Parametric Hopfield networks for classification with unbalanced data: From single to multi-task learning
Abstract: Several real-world problems nowadays are characterized by a severe imbalance in data labeling, including problems in Bioinformatics and Biomedicine, where the conclusion of numerous genome sequencing projects has generated an unprecedented amount of biological data that requires the design and the application of novel computational methods for their proper analysis and statistical/biological interpretation. In this context, the seminars present some real-world instances of unbalanced classification problems, and some state-of-the-art imbalance-aware solutions, including recently proposed classifiers based of parametric Hopfield networks (HNs). During the two seminars, unbalanced binary classification algorithms are described in two different scenarios: tasks are independent of each other; tasks are semantically related to each other. In particular, in the latter setting a recent multi-task HN model is presented. The seminar ends with some ongoing works and possible future theoretical and practical developments.
Bio: Marco Frasca is an assistant professor at Computer Science Department of the Università degli Studi di Milano from 2017. He received the Ph.D. degree in Computer Science from the Università degli Studi di Milano in 2012, and he has been a post-doc research fellow at the Biosciences and Computer Science Departments of the same university. He has been visiting researcher at the Terrence Donnelly Centre for Cellular and Biomolecular Research, University of Toronto, and at the Institute of Molecular Biology of the Johannes Gutenberg University of Mainz. He is associate editor of the International Journal of Neural Networks. He is member of the Bioinformatics Italian Society, and has been member of the International Neural Network Society. His research interests include the study of machine learning methods for unbalanced classification problem, with a particular focus on neural networks models. A main interest involves the design of machine learning techniques for emerging problems life sciences, such as protein function prediction, disease-gene prioritization, and drug-side effect prediction.
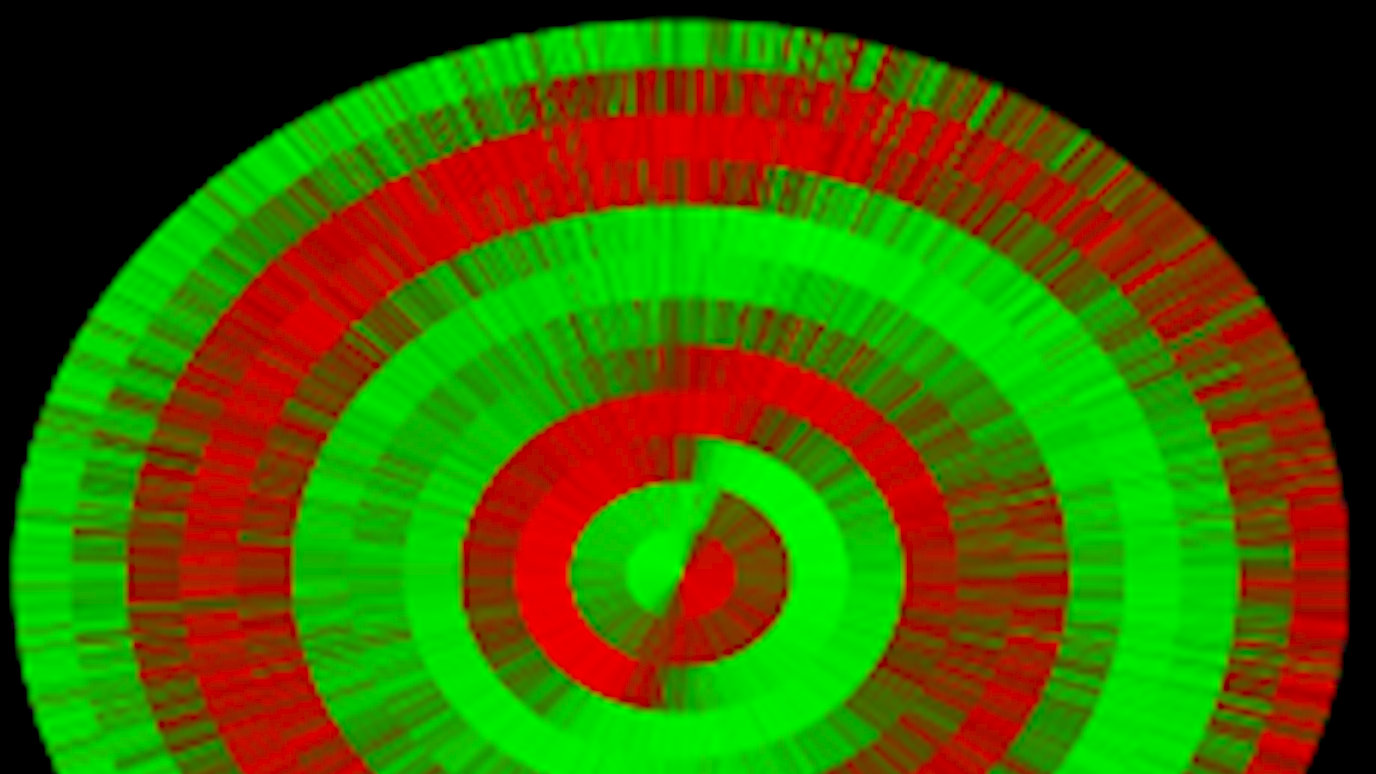